Until recently, the energy mix has been disproportionately dominated by fossil fuels which require being burnt and thus polluting our environment to produce the electric power we all need for our daily lives. In doing so, we have inadvertently selected to sacrifice our habitat for industrial and economical prosperity. To try to mitigate these negative effects and attempt to halt further contamination of the environment, significant efforts need to be made on our part. For example, to introduce and continue increasing the penetration of renewable energy sources on the electrical energy production side. It this way, electrical demand could be fulfilled based on already accessible clean energy, such as solar or wind, without harming the environment. This also applies for small island communities, such as the ones participating in the REACT project, which are usually reliant on the mainland for energy supply. This reliance often results in high energy and economic dependent on external factors, and so for achieve self-sufficiency is a crucial goal for which the increase of renewable energy generation is necessary.
However, renewable energy source production is highly dependent on meteorological conditions which are stochastic in nature, and so renewable energy production cannot be controlled – increased or decreased in accordance with the current demand. For example, demand peaks are common between 6PM and 8PM when many people come home from work. Unfortunately, this timing does not match with the highest solar radiation during the day, and therefore, peak power production from solar cells. On the other hand, the mismatch between the production and the demand side can cause instability of the electrical grid, and, consequently, the priority for finding a solution to this problem is very high. Having all this in mind, it can be concluded that careful planning and ensuring optimum energy dispatch is necessary in order to have a stable energy supply system whilst utilizing a high penetration of renewable energy sources. Therefore, estimations of the expected renewable energy production as well as user demand are required, so that energy balance planning could be carried out. Exactly this is when artificial intelligence, or more precisely, machine learning techniques, can play a significant role in platforms looking to implement different demand response programs such as REACT.
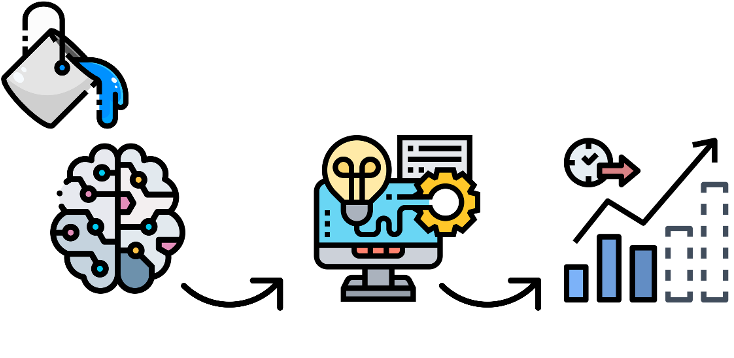
Machine learning models have a lot of numerical parameters which are adjusted in accordance with the amount of available historical data, so that their predictions are as similar to the previous real-world behavior as possible. In other words, machine learning models obtain relevant input parameters, extract relevant information from them and provides estimated output. For example, if we would like to estimate solar photovoltaic (PV) production, the first step would be to detect which factors influence its production. The most important one is definitely solar radiation since the higher solar radiation is, the higher photovoltaic production is expected to be. Furthermore, higher cloud coverage would result in lower production, and hence it should also be considered. In addition, outdoor temperature affects the efficiency of PV panels, since they are more efficient when the temperature is lower. Once all relevant input parameters are selected, the expected production can be estimated based on their values.
A similar approach could be carried out for demand forecasters, as well. One of the most common factors for the electrical load prediction are time-related parameters. As already mentioned, it is common that demand peaks are present in the early morning and afternoon during working days, whilst demand is notably lower during working hours or at night. On the other hand, the shape of the load curve during the weekends or holidays changes significantly since more users are present at home. Similarly, seasonality can also affect total energy consumption. For example, in colder climates, electrical energy is sometimes used during winter for heating purposes, whilst that is not the case during the summer. Hence, all of these conclusions are utilized during the phase of designing the ML model, so it can achieve as high precision as possible.
To sum up, as illustrated in the figure below, machine learning models detect what are the most important factors for their predictions and require a large amount of corresponding historical data. That data is further utilized by specialized algorithms which provide optimized models capable of calculating precise predictions.
In this article, we have presented some applications of machine learning in energy-related projects and tried to bring machine learning closer to the end users. However, its potential is far beyond what was presented here, so, could you think of some other applications of artificial intelligence that could be useful in the future?
Dea Pujić from Institute Mihajlo Pupin, University of Belgrade.
For creating this post following icons were used:
https://www.flaticon.com/free-icons/bucket created by justicon – Flaticon
https://www.flaticon.com/free-icons/machine-learning created by Freepik – Flaticon
https://www.flaticon.com/free-icons/arrow created by Creatype – Flaticon
https://www.flaticon.com/free-icons/machine-learning created by Eucalyp – Flaticon
https://www.flaticon.com/free-icons/prediction created by Parzival’ 1997 – Flaticon